In the vast and evolving landscape of machine learning, our journey has led us to an intriguing destination. Today, we're not exploring the intricacies of autonomous vehicles or the nuances of natural language processing. Instead, we're venturing into a domain that might sound a tad unconventional, yet is equally ripe for AI intervention. We're applying cutting-edge machine learning techniques to develop a facial recognition system for cattle and horses.
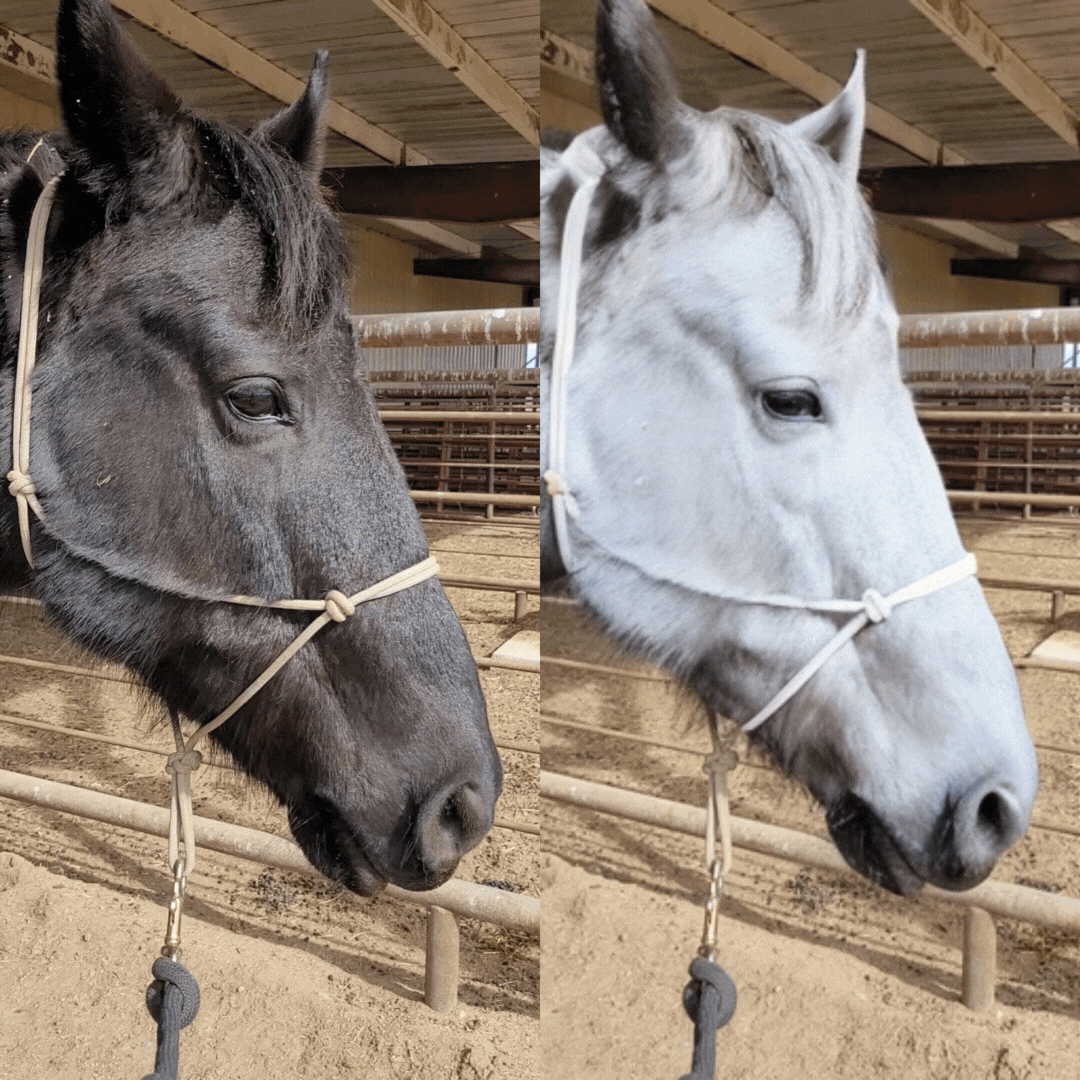
The Challenge of Data
Machine learning models are, in essence, a reflection of the data they consume. The quality, diversity, and quantity of data play a pivotal role in determining the model's performance. However, gathering a large and varied dataset, especially when it comes to cattle and horse faces, poses a formidable challenge. This is where synthetic data steps into the limelight.
Synthetic data, created algorithmically, serves as an effective substitute for real-world data. It presents a compelling solution to the data scarcity problem, allowing us to generate ample data that can be fine-tuned to encapsulate the variations and nuances we desire.
The Power Trio: Stable Diffusion, ControlNet, and DreamBooth
Our journey into synthetic data generation is spearheaded by three potent machine learning models: Stable Diffusion, ControlNet, and DreamBooth.
Stable Diffusion is an innovative deep learning model that excels in the realm of text-to-image translation. Initially developed in 2022, it transforms textual descriptions into highly detailed and accurate visual representations. Stable Diffusion also showcases a remarkable ability to perform image-to-image translations guided by a text prompt. This means we can feed an image and a textual description, like "add a white patch on the forehead", and the model would generate a modified version of the input image in line with the provided description.
ControlNet brings a game-changing twist to the narrative. It is a unique neural network structure designed to control diffusion models by introducing additional conditions. This network operates by creating two copies of the neural network blocks - a "locked" copy that preserves the original model, and a "trainable" copy that adapts based on the conditions introduced. By doing so, ControlNet ensures spatial consistency and prevents the disintegration of the original model when training with smaller datasets.
Completing the trio is DreamBooth - the model that adds the final layer of polish to our synthetic data generation process. It fine-tunes existing text-to-image models, allowing them to generate outputs that are more tailored and precise. DreamBooth's role is analogous to an artisan who meticulously refines their work to perfection.
Bridging the Gap Between Pixels and Pastures
Using these three models in harmony, we're able to generate a comprehensive synthetic dataset of cattle and horse faces. Stable Diffusion initiates the process by translating text descriptions into images. ControlNet then steps in to guide the diffusion process and ensure spatial consistency. Finally, DreamBooth refines the output, creating synthetic images that closely mirror the diversity and complexity of real-world livestock faces.
And that's how we're navigating the intricate dance of synthetic data generation for building a facial recognition system for cattle and horses. It's a fascinating blend of machine learning, creativity, and a touch of the unexpected. Stay tuned as we continue to push the boundaries of what AI can do, exploring uncharted territories, and transforming the way we interact with the world around us.